Banks and fraudsters are engaged in a never-ending game of cat and mouse. On one side, fraudsters move money around to remove traces of criminality. On the other, banks are on the lookout for suspicious activity that indicates financial fraud.
“Criminals put money through the financial system in a series of layers to mask its original source, getting to a point where that money is cleaned and can be used and integrated into the financial system for any kind of purchase or investment,” says Livia Benisty, chief business officer and former global head of AML at, Banking Circle – a payments bank that is pioneering the use of AI in AML.
Money laundering regulations require banks and financial services to demonstrate methods for spotting this behaviour.
The stakes are high for financial firms. Failure to maintain adequate anti-money laundering (AML) controls can lead to multi-million-pound fines.
For a smaller private bank – with say, 150 clients doing a couple of transactions a week – an analyst could in theory look through the transactions manually on a spreadsheet and flag suspicious behaviour.
But with larger financial firms processing hundreds of thousands – or even millions – of transactions every day, spotting suspicious activity using manual processes would be like spotting a needle in a haystack.
That’s why artificial intelligence (AI) is increasingly being embraced by banks and financial companies to monitor and prevent fraud at scale. Indeed, Banking Circle has developed a hybrid solution that combines the best of AI, machine learning (ML) and the traditional rules-based approach to all transactions Banking Circle handles on behalf of clients.
From basic automation to AI in AML
AI in AML traces its roots back to basic rules-based systems. Banks would set up an automated fixed rule that might indicate suspicious behaviour, such as a threshold for a payment size.
“Banks would have a different rule for an individual than they would for a company like Coca-Cola, which is essential because most people and companies have very different spending patterns and volumes,” explains Benisty.
For decades, banks have been using basic rule-based technology to flag suspicious financial activity. Over time, these rules have become more complex, enabling a more targeted approach to AML.
“You would segregate your client base into different types of rules,” explains Benisty. “And those rules could be things like the amount spent within a given period, or the number of transactions within a given period. It could even be the types of institutions or companies or people that financial institutions expect clients to interact with.”
However, even the more advanced static rules have flaws, characterised by fixed, narrow parameters that meant consumers had to notify their bank of travel abroad to avoid their account from being blocked.
“And it was utterly pointless, because it still blocked your card and you’d still have to spend time and money calling customer service at 4am London time because you were stuck,” says Benisty. “So that’s what happens when static rules go wrong, when they’re too binary.”
Further underlining the problems facing today’s basic AML systems, one report puts the false positive rate at 95%. This is when a legitimate transaction is flagged as potentially suspicious, when it is actually clean, highlighting inefficiencies within the industry.
More advanced AI
The more advanced AML systems that are increasingly being adopted by financial firms rely heavily on AI and machine learning to detect complex patterns.
Instead of a static transaction threshold, an AI model can look at a customer’s spending patterns over a rolling period across multiple variables simultaneously.
“Rather than just looking at one metric that indicates risk in your defences, an AI-based AML system is able to pick up on other characteristics of a payment, which could indicate a greater likelihood of suspicious activity,” explains Benisty. “That’s the next level – where you’re not just measuring against one metric, but a series of indicators, which with certain weighting would be more likely to constitute suspicious behaviour.”
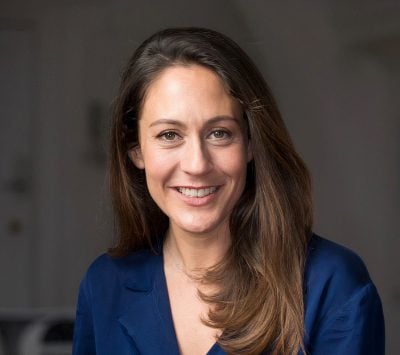
The series of indicators that point to suspicious activity would not be specifically defined by the analyst from the outset; the system would be able to identify outliers, or activity that looks more like those alerts which have previously generated escalations or suspicious activity filings.
Benisty says that AI has a “much better grasp of the data” to detect patterns that a human would be unable to spot, due to the volume and complexity of the transactions. Without AI or machine learning, banks are “pretty reliant” on static rules.
While there’s plenty of hype around AI, Benisty has a more grounded view of its benefits and limitations.
“What AI can do is start to look for the different characteristics of those payments that are going to the higher level and getting escalated – and therefore more likely to indicate suspicious behaviour,” she says.
AI in AML adoption
Most of the bigger banks are already using AML AI tools to rank transaction alerts by risk, then auto-clear things below a certain risk level.
But despite the advantages of AI in AML, there are hurdles to the adoption of the more advanced tools, from understanding procurement processes to implementing the solution.
One common challenge, says Benisty, is a fear of how regulators will react if a company adopts AI solutions, due to a fear that they will be unable to explain how the AI comes to its decisions.
“I think there’s a bit of reticence there. I think there’s a belief that because AI is so hyped up it’s like a panacea solution for everything”.
However, companies don’t need to dive in at the deep end – they can start with basic AI and introduce more rules over time. And for banks, there is an opportunity to embrace AI in AML as part of an existing digital transformation project.
Plus, regulators are becoming better educated in AI systems in regtech, which Benisty expects to drive further adoption.
Perhaps unsurprisingly, newer fintech companies are better positioned to embrace AI AML tools as they’re not bogged down by the same legacy tech stacks.
“It’s a lot easier than going into a 200-year-old institution that was largely built through mergers and acquisitions. It’s not even that their data is messy – it’s the five other companies they’ve acquired that have messy data, often across multiple geographies and in different data formats such as paper documents,” explains Benisty.
However, it is in the larger banks that have significantly higher transaction volumes where AI has the most value.
“What we’ve seen over the course of the last year, through various forms of data analysis, including the use of our own AI rules, is we have doubled the number of payments and maintained the number of alerts at a static level,” says Benisty. “So effectively, the hit rate has improved.”
Predicting fraudulent behaviour with AI?
As banks increasingly adopt AI to monitor and prevent financial fraud, where next for the technology?
For Benisty, it’s heading towards predictive models, where patterns of activity reveal new ways that criminals might engage in fraudulent activities.
This could see banks get one step ahead in the eternal game of cat and mouse.
“Through AI, we detect typology – so patterns are behavioural analysis,” says Benisty. “Based on these patterns, we make informed decisions to catch criminal activity. We’ve proven this is a successful method, yet, once you’ve uncovered a pattern, the criminals have moved on; you can still be several years out from it ever being used again. It’s quite backwards looking. Ideally, we want to get to a point where it can spot a new pattern in real time, and that’s really exciting.”
Current systems are still largely reliant on learning from what criminals have done in the past, plus, uncovering new criminal methods is still largely reliant on criminals messing up. Benisty gives the example of drug traffickers getting caught after one gang member sent an image of his dog over a messaging app. Police zoomed in on the dog’s tag, which showed the gang member’s phone number.
Will criminals be able to change tactics and avoid future predictive AI models? Benisty thinks they will.
“I don’t see this ever stopping. I think criminals will always find a way. Not to mention there will always be cash,” she says.
“But I think the key here is to get faster at uncovering new patterns of behaviour, get better at identifying where real risk lies – not where the perceived risk lies. And in the meantime, it’s about clearing out the noise so that financial firms aren’t paying however many analysts to sit there and get rid of level one hits that they are bored to death of.”
In paid partnership with Banking Circle, the tech-led payments bank.
The post How AI can transform transaction monitoring and prevent financial fraud appeared first on UKTN | UK Tech News.